Alarms Engine
The Alarms Engine helps you stay informed about the availability and performance of your monitored resources. There are different ways in which Site24x7 identifies and alerts on anomalies in the behavior of a monitored resource. However, the Alarms Engine is the mechanism that decides the severity of an alert. Sometimes, an anomaly may self-rectify without a user's intervention. In such instances, monitors should not raise false alerts, which the Alarms Engine prevents.
Status detection
Monitors check the resources periodically to record data, and when they breach a configured threshold level, the monitors are declared down, trouble, or critical.
For instance, in the case of a website monitor, when a monitor detects downtime, depending on the alert settings, notifications are triggered when a monitor is down—either immediately or after verifying the issue across multiple locations. You can also override these settings to receive alerts only after a monitor has been down for a specified number of consecutive polls. Consecutively, the Alarms Engine triggers alert. Monitoring continues to identify any change in status, and Site24x7 notifies the relevant stakeholders of the change.
Threshold and availability
The status of a resource is set as up, down, trouble, or critical based on the data from its Threshold and Availability profile. The two different types of threshold settings are:
- Static threshold
- Zia-based threshold
Customize what alerts you want to receive, when you want to receive them, and how you receive them using On-Call Schedule, Attribute Alerts Group, Alarms Category, and Notification Profile.
Static thresholds
Users or admins can set threshold parameters for alerts. These threshold parameters may vary depending on the monitor type. For each metric, you can define a threshold manually, and when this threshold is breached, an alert is triggered.
Zia-based thresholds
Zia-based thresholds involve ML-powered models setting dynamic thresholds after understanding the system. Here, ML studies the system for a set of period, say 15 days, and trains the model. The trained model can automatically set a threshold value depending on the benchmark performance of the resource, and when it exceeds the threshold, it alerts you.
The threshold set by Zia varies for different monitors based on their behavioral patterns. Whenever there is a change in the functionality of the resource, Zia retrains the model based on the changes. When there is a sudden spike or dip from the threshold, Zia-based anomaly detection alerts you about the unusual behavior. With timely notice, you can take immediate measures to avert downtime and harness resources effectively.
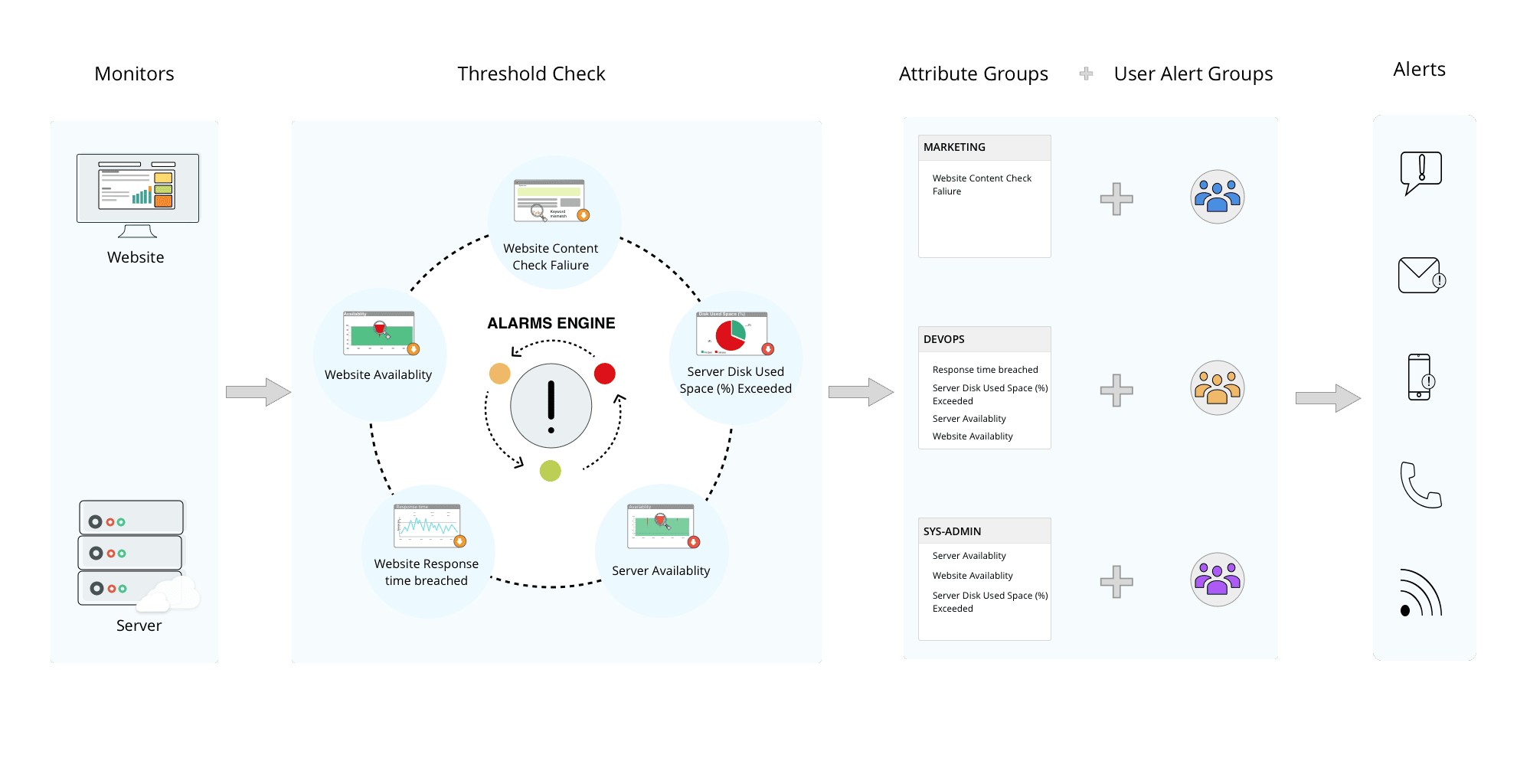
After downtime, a root cause analysis report is automatically sent to you through the configured alert medium to mitigate the mean time to repair.